Security Monitoring – It’s All About the Data
September 11, 2018 |
Security
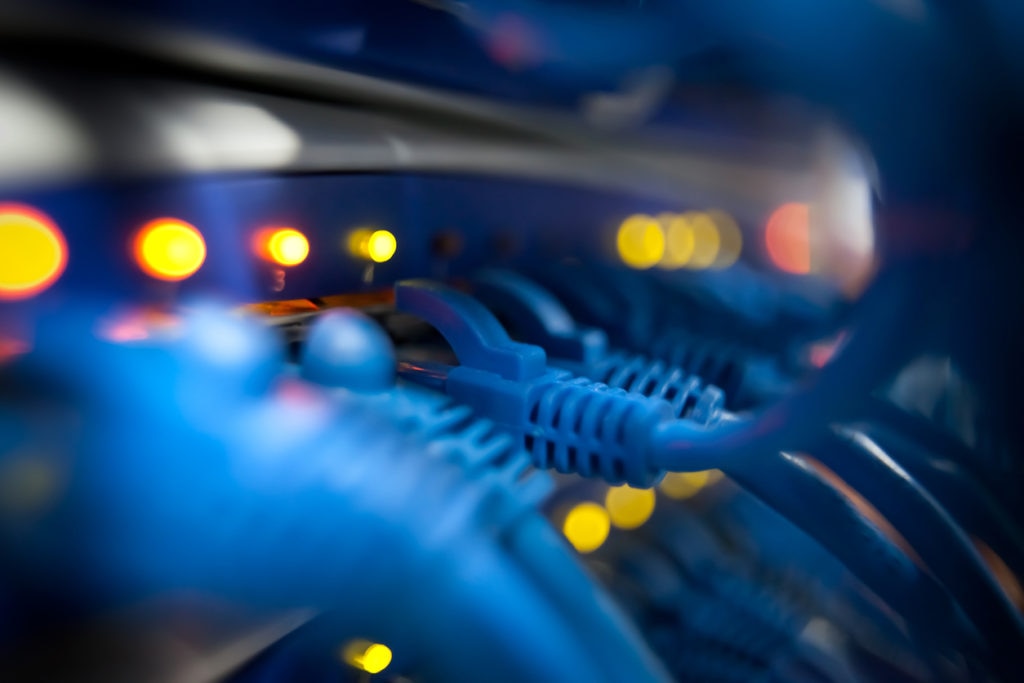
In this series, we’ve covered some key areas that can help prepare for potential attacks. Preparation is essential. Security policies are essential. Understanding your network and its assets is essential. What happens if a threat is detected? What can we do to monitor for threats? This final blog will look at security monitoring through an understanding of data. Data contains information and exposes actions. Data is the vehicle for compromise, so it is dynamic and must be tracked in real time. Being able to understand data streams is important for identifying and reacting to threats and then applying the correct protection and mitigation methods. Investigation and response depends on an understanding of these data types.
Raw data: Sourced directly from a host in the creation of an event log. Some events are pushed from the source using protocols such as syslog and SNMP. Protocols such as SCP, SFTP, FTP, and S3 are typically used to pull event logs from a source system.
Parsed Data: Parsing involves matching raw logs to rules or patterns to determine which text strings and variables should be mapped to database fields or attributes. This is a common function of SIEM tools that aggregate raw data streams for a wide variety of telemetry types. Sometimes an agent is used on a source host or an intermediate aggregation point to map raw message data into a vendor specific format such as CEF Syslog or LEEF, which is a first step to normalizing data.
Normalized Data: Normalization means transforming variables in the data to a specific category or type for aggregation purposes. For example, taking several attributes that refer to a threat type using various naming conventions and assigning them to a specific attribute as defined in the processing system’s schema. This introduces efficiency when storing and searching data.
Full Packet Capture: A capture of all Ethernet/IP activity in contrast to filtered packet capture focusing on a subset of traffic. Important for network forensics or cybersecurity purposes, especially in the case of an advanced persistent threat whose characteristics may be missed in a filtered capture. FPC may be used in static and dynamic analysis systems or detonated in a sandbox for greater understanding.
Metadata: Summary information about data. Extracted from FPC to provide a focus on key fields and values not just payloads, which may be encrypted. By looking at the metadata associated with a flow of network traffic, it can be easier to tell the difference between legitimate and bad traffic rather than trying to examine the detailed contents of every data packet. Important metadata include transaction volumes, IP addresses, email addresses, and certificates for TLS and SSL.
Flow Data: Flows represent network activity in a session between two hosts by normalizing IP addresses, ports, byte and packet counts, and other data, into flow records. A flow starts when a flow collector detects the first packet that has a unique source IP address, destination IP address, source port, destination port, and other specific protocol options. Often used to look for threats identifiable by their behavior across a flow rather than through atomic actions.
DPI Data: Deep packet inspection is stateful packet classification up to the application layer usually carried out as a function of next-generation firewalls and IPS. An expansion of the traditional "5-tuple:" source and destination IP, source and destination port, and protocol. DPI is part of Application Visibility and Control (AVC) systems that extract useful metadata and compare it to the well-known behaviors of applications and protocols to identify anomalies and statistically significant deviations in those behaviors.
Statistical Data: Makes use of statistical normalization where the values of columns in a dataset are changed to use a common scale, without distorting differences in the ranges of values or losing information. It is required for some algorithms to model the data correctly such as curve fitting algorithms like the clustering algorithms used in Unsupervised Machine Learning. Statistical data is used to detect user-based threats with user and entity behavior analytics or to identify network threats through network traffic and behavior analysis.
Extracted Data: Data retrieved from data sources (like a SIEM database) using specific search patterns to correlate events to build a complete picture of a session or attack. For example, Mapping DNS logs and HTTP logs together to find a threat actor by searching on metadata or IoCs, or tracking the path of email using the Message ID (MID) value.
Security Intelligence Enriched Data: Adds information such as reputation and threat scores to metadata to help identify potentially compromised hosts within the network based on a threat analysis report containing malicious IP address or domains, for example Mapping DNS, HTTP, and threat intelligence data together to identify connections to known blacklisted sites.
That’s a wrap on this series presenting some cybersecurity fundamentals. Remember, you can’t plan for every threat, and you can’t anticipate the actions of users – both friend and foe. What you can do is be a prepared as possible and reduce the time to detect associated with attacks. You can also put processes and knowledge in place to efficiently respond and remediate. Know your environment and keep up to date with the changes in the threat landscape and how they relate to your use cases. Don’t get complacent—stay prepared.